StyleFaceV: Face Video Generation via Decomposing and Recomposing Pretrained StyleGAN3
2The Chinese University of Hong Kong
3SenseTime Research
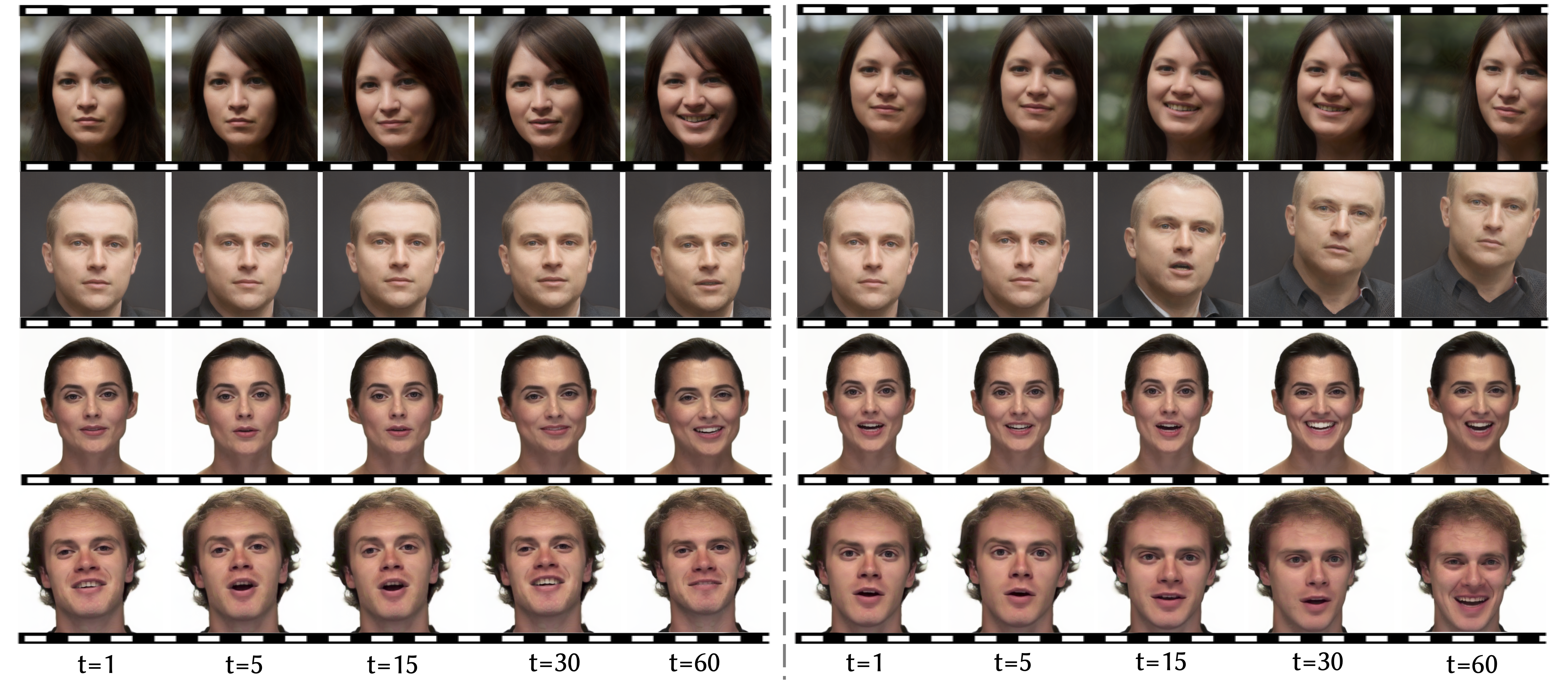
Abstract
Realistic generative face video synthesis has long been a pursuit in both computer vision and graphics community. However, existing face video generation methods tend to produce low-quality frames with drifted facial identities and unnatural movements. To tackle these challenges, we propose a principled framework named StyleFaceV, which produces high-fidelity identity-preserving face videos with vivid movements. Our core insight is to decompose appearance and pose information and recompose them in the latent space of StyleGAN3 to produce stable and dynamic results. Specifically, StyleGAN3 provides strong priors for high-fidelity facial image generation, but the latent space is intrinsically entangled. By carefully examining its latent properties, we propose our decomposition and recomposition designs which allow for the disentangled combination of facial appearance and movements. Moreover, a temporal-dependent model is built upon the decomposed latent features, and samples reasonable sequences of motions that are capable of generating realistic and temporally coherent face videos. Particularly, our pipeline is trained with a joint training strategy on both static images and high-quality video data, which is of higher data efficiency. Extensive experiments demonstrate that our framework achieves state-of-the-art face video generation results both qualitatively and quantitatively. Notably, StyleFaceV is capable of generating realistic 1024 × 1024 face videos even without high-resolution training videos.
Video
Sampling diverse appearances and motions
StyleFaceV (ours)
Random Results on RAVDESS
StyleFaceV (ours)
DI-GAN
SG-V
Random Results on FFHQ
StyleFaceV (ours)
MCHD-pre
MCHD-re
Citation
@misc{https://doi.org/10.48550/arxiv.2208.07862, doi = {10.48550/ARXIV.2208.07862}, url = {https://arxiv.org/abs/2208.07862}, author = {Qiu, Haonan and Jiang, Yuming and Zhou, Hang and Wu, Wayne and Liu, Ziwei}, keywords = {Computer Vision and Pattern Recognition (cs.CV), FOS: Computer and information sciences, FOS: Computer and information sciences}, title = {StyleFaceV: Face Video Generation via Decomposing and Recomposing Pretrained StyleGAN3}, publisher = {arXiv}, year = {2022}, copyright = {arXiv.org perpetual, non-exclusive license} }